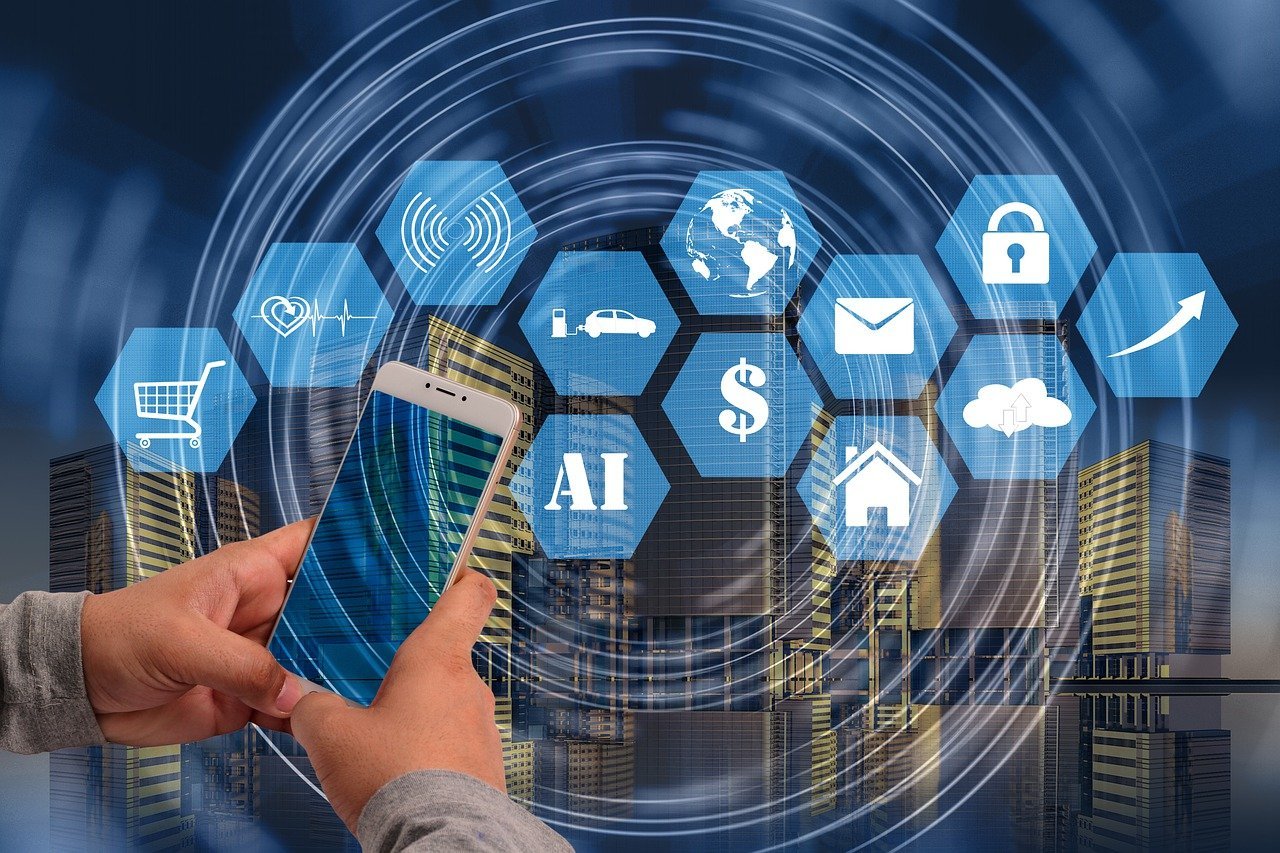
Behind-the-Scenes of Agentic AI Development: Key Challenges and Breakthroughs
Agentic AI is revolutionizing technology by enabling systems to act independently, make decisions, and adapt in real time.
While the end results of this technology are often showcased—think autonomous vehicles, adaptive chatbots, and self-managing systems—much of the behind-the-scenes work that makes these capabilities possible remains hidden.
Developing Agentic AI is far from straightforward; it involves overcoming technical challenges, addressing ethical concerns, and achieving breakthroughs in autonomy and adaptability.
In this blog, we’ll take a behind-the-scenes look at how Agentic AI is developed, highlighting the major hurdles researchers face and the breakthroughs that are pushing the boundaries of what autonomous systems can achieve.
What is Agentic AI?
Agentic AI refers to artificial intelligence systems that can operate autonomously, set goals, and make decisions to achieve those goals.
These systems go beyond simple task automation by:
- Adapting to their environment: Reacting to real-time changes dynamically.
- Solving problems independently: Without needing human intervention.
- Collaborating effectively: Particularly in multi-agent environments where multiple autonomous systems interact.
For example, Agentic AI in logistics might reroute shipments autonomously to avoid delays caused by traffic, weather, or unforeseen disruptions—without waiting for human instructions.
Key Challenges in Developing Agentic AI
Balancing Autonomy and Control
One of the biggest challenges in Agentic AI development is striking the right balance between autonomy and control. Systems need the freedom to make decisions but must still operate within ethical and practical constraints.
- Challenge: How do you ensure autonomous systems don’t act in ways that conflict with human intentions or safety?
- Solution: Developers embed “rules of engagement,” such as ethical guidelines and safety protocols, while retaining the ability for human intervention if needed.
Context Awareness
Agentic AI systems must understand and adapt to their environment. This requires sophisticated sensors, data interpretation, and decision-making models.
- Challenge: Interpreting complex, unstructured environments like crowded streets or changing market conditions.
- Solution: Dynamic context modeling, which uses real-time data from multiple sources to guide decision-making. For example, self-driving cars rely on lidar, cameras, and GPS to interpret road conditions and adjust accordingly.
Predicting and Managing Emergent Behaviors
When multiple autonomous agents interact, they can exhibit emergent behaviors—unexpected outcomes that arise from their collaboration.
- Challenge: Ensuring that these emergent behaviors are beneficial rather than disruptive.
- Solution: Developers use simulated environments to monitor and refine how agents interact, testing scenarios where collaboration leads to efficiency rather than chaos.
Ethical Decision-Making
Autonomous systems must make decisions that align with human values, especially in high-stakes applications like healthcare or autonomous vehicles.
- Challenge: Teaching AI systems to weigh complex ethical dilemmas, such as prioritizing safety during unavoidable accidents.
- Solution: Embedding ethical decision frameworks and enabling systems to explain their reasoning. Explainable AI (XAI) ensures transparency and accountability in autonomous decision-making.
Data Efficiency and Scalability
Agentic AI systems often require vast amounts of training data to function effectively. Collecting and processing this data can be resource-intensive.
- Challenge: Reducing data dependency without compromising performance.
- Solution: Advances in unsupervised and self-supervised learning enable agents to learn patterns and adapt with minimal labeled data.
Breakthroughs Driving Agentic AI Forward
Hierarchical Reinforcement Learning (HRL)
Traditional reinforcement learning has been a cornerstone of Agentic AI, but HRL takes it a step further by enabling systems to break down complex goals into smaller, manageable tasks.
- Breakthrough: HRL allows autonomous systems to pursue long-term objectives while optimizing their performance on subtasks.
- Example: A warehouse robot with HRL might plan its overall objective of organizing inventory while deciding the most efficient routes to transport items.
Simulation-Based Training
Developers are leveraging advanced simulation environments to train AI systems. Simulated environments allow agents to encounter countless scenarios, from rare edge cases to everyday tasks, without real-world risks.
- Breakthrough: Sim-to-real transfer technology ensures that knowledge learned in simulations translates seamlessly into real-world applications.
- Example: Drones trained in simulated disaster scenarios are now being used for real-world rescue missions.
Multi-Agent Coordination
One of the most exciting breakthroughs is in enabling multiple AI agents to work together toward a shared goal. This is particularly valuable in logistics, defense, and disaster response.
- Breakthrough: Advances in communication protocols allow agents to share information and make collective decisions without central control.
- Example: Autonomous robots in a warehouse collaborate to efficiently move items while avoiding collisions.
Advances in Edge AI
Edge AI allows systems to process data locally rather than relying on cloud-based servers. This reduces latency and enables real-time decision-making in environments where connectivity may be limited.
- Breakthrough: Edge AI is making autonomous systems faster, more reliable, and less dependent on centralized infrastructure.
- Example: Self-driving cars with edge AI can make split-second decisions even if network connectivity is lost.
Behind-the-Scenes Challenges with Deployment
Even with technical breakthroughs, deploying Agentic AI in real-world scenarios comes with its own set of challenges:
Real-World Testing
Simulations can’t replicate every possible scenario, so real-world testing is crucial. This often reveals unexpected issues, from edge cases to environmental variables.
Building User Trust
End users must trust autonomous systems, especially in fields like healthcare or finance. Building transparency into AI behavior is critical for adoption.
Scalability Across Industries
Scaling Agentic AI from niche applications to widespread adoption requires addressing industry-specific constraints, such as regulatory hurdles and cost barriers.
Real-World Applications of Agentic AI
Healthcare
- Breakthrough Application: AI systems that autonomously monitor ICU patients, predict complications, and alert medical staff when interventions are needed.
- Impact: Reduces workloads for healthcare providers and improves patient outcomes.
Supply Chain Management
- Breakthrough Application: Autonomous systems that dynamically reroute shipments based on real-time traffic and weather data.
- Impact: Reduces costs, enhances efficiency, and minimizes delays.
Disaster Response
- Breakthrough Application: Autonomous drones map disaster zones, identify survivors, and deliver critical supplies.
- Impact: Accelerates response times and saves lives.
Energy Management
- Breakthrough Application: AI agents autonomously balance power distribution in renewable energy grids, adapting to changing supply and demand.
- Impact: Enhances energy efficiency and sustainability.
Looking Ahead: What’s Next for Agentic AI?
As the field evolves, the development of Agentic AI will focus on:
- Human-AI Collaboration: Creating systems that augment human capabilities rather than replacing them.
- Universal Ethics: Developing global frameworks to guide ethical AI behavior.
- Self-Evolving Systems: Enabling AI systems to adapt and improve without human intervention.
Behind every autonomous action lies a complex web of innovation, experimentation, and problem-solving.
By overcoming these challenges and embracing breakthroughs, Agentic AI will continue to redefine how technology operates—turning machines into collaborators and partners in solving the world’s most complex problems.